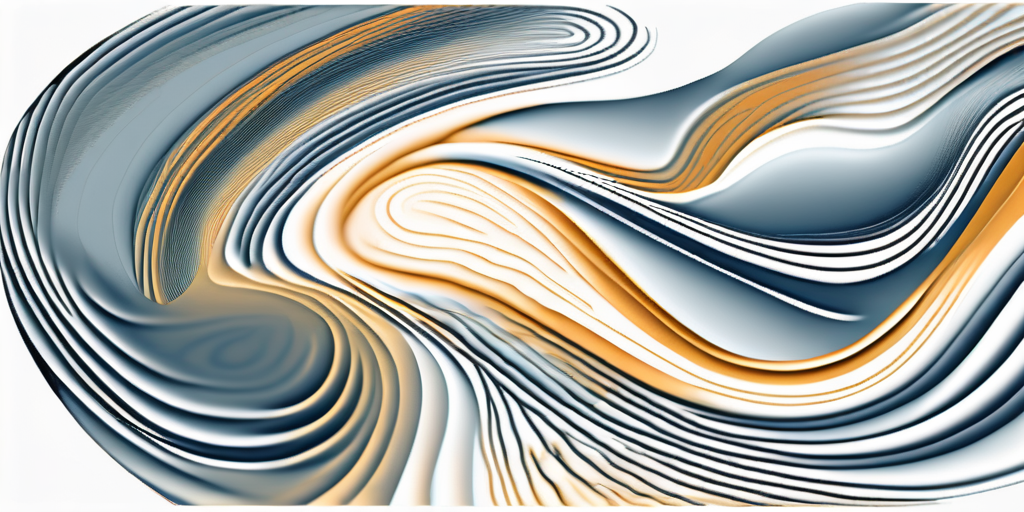
Introduction to Detached Eddy Simulation (DES)
What is Detached Eddy Simulation (DES)?
Detached Eddy Simulation (DES) is a computational fluid dynamics (CFD) technique that has gained popularity in recent years. It was developed as an alternative to the traditional Reynolds-Averaged Navier-Stokes (RANS) method to better capture the effects of turbulence in complex flow phenomena. In this article, we will explore the challenges that turbulence poses to CFD and why DES was needed as an alternative to RANS. Furthermore, we will discuss how DES adds time-dependence back into the RANS equations, improving the accuracy of simulations.
The Challenge of Turbulence to CFD
Turbulence is a ubiquitous phenomenon in fluid flow that occurs when the velocity and pressure of the fluid exhibit irregular and chaotic behavior. It is a challenging aspect for Computational Fluid Dynamics (CFD) simulations, as it introduces significant complexity to the equations governing fluid dynamics.
Turbulent flows are characterized by vortices, eddies, and fluctuations in velocity and pressure, making them difficult to model accurately using simplified mathematical approaches. The intricate nature of turbulence poses a great challenge to engineers and scientists who seek to understand and predict the behavior of fluid flows in various applications.
The traditional Reynolds-Averaged Navier-Stokes (RANS) method, which averages the governing equations over time, was widely used in CFD simulations to simulate turbulent flows. However, RANS was found to be limited in its ability to capture the unsteady nature of turbulence, as it assumes the flow to be steady. This limitation led to the development of alternative methods such as Detached Eddy Simulation (DES).
DES combines the advantages of both RANS and Large Eddy Simulation (LES) methods. It uses RANS equations in the near-wall regions, where the flow is typically more predictable and less turbulent, and switches to LES equations in the core flow regions, where the turbulence is more dominant. This hybrid approach allows for a more accurate representation of the flow physics, capturing both the large-scale turbulent structures and the smaller-scale turbulent fluctuations.
Another approach to tackling turbulence in CFD simulations is the use of Reynolds Stress Models (RSM). RSMs aim to capture the anisotropic nature of turbulence by explicitly modeling the Reynolds stresses, which represent the correlations between the fluctuating velocity components. By incorporating additional transport equations for the Reynolds stresses, RSMs provide a more detailed description of the turbulent flow field.
Furthermore, the advent of high-performance computing has enabled the use of Direct Numerical Simulation (DNS) for studying turbulence. DNS solves the Navier-Stokes equations directly, without any turbulence modeling assumptions. This approach provides the most accurate representation of turbulence but is computationally expensive and limited to relatively low Reynolds numbers due to the high resolution requirements.
With the continuous advancements in computational power and numerical algorithms, researchers are constantly striving to improve the accuracy and efficiency of turbulence modeling in CFD. Various turbulence models and simulation techniques are being developed and refined to better capture the complex dynamics of turbulent flows. These advancements not only enhance our understanding of turbulence but also have practical implications in industries such as aerospace, automotive, and energy, where accurate predictions of fluid flow behavior are crucial for design optimization and performance evaluation.
Why an Alternative to RANS was Needed
While RANS (Reynolds-Averaged Navier-Stokes) is effective in simulating steady-state flows, its shortcomings become evident when dealing with flows that exhibit unsteady behavior, such as flows over complex geometries or with strong vortices and swirling motion. RANS fails to capture the unsteady fluctuations and does not provide an accurate representation of the flow phenomena.
In order to overcome these limitations, researchers and engineers sought to develop an alternative method that could accurately simulate both steady and unsteady flows. This led to the development of Detached Eddy Simulation (DES), which combines the strengths of both RANS and Large Eddy Simulation (LES).
LES is a computationally expensive method that explicitly resolves the large-scale turbulent eddies but models the small-scale turbulence. It provides a detailed representation of the flow dynamics by directly simulating the large-scale structures. However, LES alone is not suitable for simulating steady flows due to its high computational cost.
By incorporating time-dependence back into the equations, DES is able to capture both the steady and unsteady components of the flow, resulting in more accurate predictions. In regions where the flow is predominantly steady, RANS equations are used, while in regions with unsteady behavior, LES equations are employed. This hybrid approach allows for a more efficient and accurate simulation of complex flows.
One of the key advantages of DES is its ability to capture the flow phenomena near solid boundaries. RANS models often struggle to accurately predict the flow behavior in these regions, especially in the presence of complex geometries. DES, on the other hand, is able to resolve the flow structures near the boundaries, providing a more realistic representation of the flow.
Another advantage of DES is its ability to handle flows with strong vortices and swirling motion. These types of flows are commonly encountered in various engineering applications, such as aerodynamics and combustion. RANS models often fail to capture the intricate details of these flow features, leading to inaccurate predictions. DES, with its ability to resolve large-scale eddies, is better equipped to simulate such flows and provide more reliable results.
Despite its advantages, DES does have some limitations. The computational cost of DES is higher compared to RANS due to the inclusion of LES equations. This makes DES more computationally demanding and may limit its application to certain cases where computational resources are limited.
In conclusion, the development of DES as an alternative to RANS has greatly improved the accuracy and reliability of flow simulations, particularly for flows with unsteady behavior and complex flow phenomena. By combining the strengths of RANS and LES, DES is able to provide a more comprehensive understanding of the flow dynamics, making it a valuable tool in various engineering fields.
Adding Time-Dependence Back into the RANS Equations with DES
The main idea behind DES is to perform RANS calculations in regions of the flow where turbulence is relatively smooth and predictable, while switching to LES in regions where the turbulence is more complex and unsteady. This approach allows for a better representation of both the mean flow and the turbulent fluctuations.
DES achieves this by introducing a criterion to identify the transition point between RANS and LES regions. This criterion, often referred to as the turbulence length scale criterion, determines the scale at which the flow becomes unsteady. When the flow reaches this critical scale, DES seamlessly transitions from RANS to LES, resolving the larger turbulent structures while modeling the smaller-scale turbulence.
The time-dependent part of DES involves solving the RANS equations in a transient manner, allowing the flow to evolve over time. This time dependency brings an additional level of accuracy to the simulations, as it captures the unsteady features of the flow. By reintroducing time dependence into the RANS equations, DES improves upon the limitations of traditional RANS and provides a more realistic representation of the flow behavior.
Conclusions
Detached Eddy Simulation (DES) is a computational fluid dynamics technique that addresses the challenges posed by turbulence to CFD simulations. By combining the strengths of RANS and LES, DES is able to capture both the steady and unsteady components of turbulent flows, resulting in more accurate predictions. DES adds time-dependence back into the RANS equations, allowing for a better representation of the complex and chaotic nature of turbulence. As CFD continues to advance, the adoption of methods like DES will pave the way for more accurate and reliable simulations of turbulent flow phenomena.