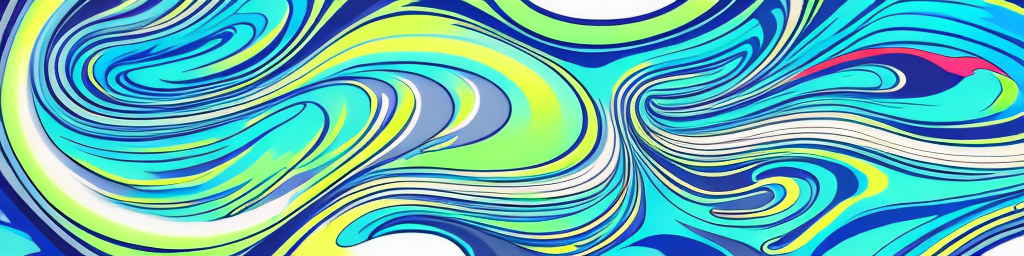
Introduction to CFD Turbulence Models: Understanding, Types, and Applications
What Is a Turbulence Model and Why do I Need One?
Turbulence is a ubiquitous phenomenon in the field of fluid dynamics. Turbulence modeling aims to provide predictive tools for the behavior of fluids in various applications. In this article, we will delve into the basics of turbulence modeling, its development over time, the different types of models, and when and why to use them.
A Model Within a Model - Small Scale Turbulence
Large-scale turbulence models have become an essential tool in various industries such as aerospace, automotive, and marine engineering. These models allow engineers to predict the behavior of fluid flow in complex systems and optimize designs to improve performance. However, predicting the behavior of small-scale turbulence poses a significant challenge.
Small-scale turbulence is a phenomenon that occurs at a much smaller length scale than the large-scale turbulence that is typically modeled. It is crucial in understanding the detailed flow behavior of fluids, and it substantially affects the overall characteristics of the fluid flow. Small-scale turbulence is responsible for the mixing of fluids, the transfer of heat and mass, and the dissipation of energy in the fluid flow.
Performing a direct numerical simulation to model small-scale turbulence is computationally expensive and requires enormous computational power. Direct numerical simulation involves solving the Navier-Stokes equations, which describe the motion of fluids, at a very high resolution. This approach is not feasible for most engineering applications due to the large number of computational resources required.
Instead, researchers have developed models that account for small-scale turbulence in a coarse-grained model of the fluid flow. These models are based on the idea of subgrid-scale modeling, which involves modeling the small-scale turbulence as a separate entity within the larger-scale turbulence model. This approach allows engineers to capture the effects of small-scale turbulence without the need for direct numerical simulation.
One popular subgrid-scale model for small-scale turbulence is the Smagorinsky model. This model is based on the assumption that the small-scale turbulence behaves like a viscous fluid. The Smagorinsky model uses a constant coefficient to estimate the energy dissipation rate of the small-scale turbulence. This coefficient is determined by fitting the model to experimental data.
Another approach to modeling small-scale turbulence is the Large Eddy Simulation (LES) model. LES involves solving the Navier-Stokes equations for the large-scale turbulence and modeling the small-scale turbulence as a separate entity. The LES model is computationally less expensive than direct numerical simulation but more expensive than the Smagorinsky model. However, the LES model provides a more accurate representation of the small-scale turbulence than the Smagorinsky model.
In conclusion, small-scale turbulence is a crucial phenomenon in understanding the behavior of fluid flow. Modeling small-scale turbulence poses a significant challenge due to its high computational cost. However, subgrid-scale modeling provides a viable approach to capturing the effects of small-scale turbulence in a coarse-grained model of the fluid flow. The Smagorinsky model and the Large Eddy Simulation model are two popular subgrid-scale models for small-scale turbulence.
Turbulence Model Development Time Line
The development of turbulence models is a fascinating and ongoing field of research that has its roots in the early 20th century. Researchers in various industries began investigating the behavior of fluids, and as they did so, they realized that there were complex phenomena that they could not fully account for with existing models.
As computational power increased, researchers were able to develop more sophisticated models that could account for more complex phenomena. One of the most significant developments in this regard was the creation of Reynolds stress models, which allowed researchers to account for the effects of turbulence on the mean flow. This was a major breakthrough, as it allowed researchers to better understand the behavior of fluids in a wide range of applications.
Another major development in the field of turbulence modeling was the creation of Large Eddy Simulation (LES). This technique involves simulating the large-scale structures of turbulence, while modeling the smaller scales using subgrid-scale models. LES has proven to be a valuable tool in a wide range of applications, including aircraft design, weather forecasting, and combustion modeling.
Detached Eddy Simulation (DES) is another important development in the field of turbulence modeling. This technique combines elements of Reynolds-averaged Navier-Stokes (RANS) and LES models to simulate both the large and small scales of turbulence. DES has proven to be particularly useful in simulating complex flows, such as those found in wind turbines and aircraft engines.
Today, researchers continue to refine turbulence models and develop new methods to account for previously unmodeled phenomena. One area of active research is the development of models that can account for the effects of turbulence on heat transfer. This is a particularly important area of research, as it has implications for a wide range of applications, including combustion engines and nuclear reactors.
Another area of active research is the development of models that can account for the effects of turbulence on chemical reactions. This is an important area of research, as it has implications for a wide range of applications, including combustion modeling and atmospheric chemistry.
In conclusion, the development of turbulence models is an ongoing and fascinating field of research that has led to a better understanding of the behavior of fluids in a wide range of applications. As computational power continues to increase, researchers are able to develop more sophisticated models that can account for more complex phenomena, and this is leading to exciting new developments in the field.
How to Choose the Right Turbulence Model
Choosing the correct turbulence model for a specific application is essential to obtain accurate results. The choice depends on factors such as the flow regime, required accuracy, geometry, and computational resources. The most straightforward turbulence models are the zero-equation models or the one-equation models. However, they may not provide accurate results for complex flows. Two-equation models such as the k-epsilon and k-omega models are the most commonly used turbulence models for general-purpose applications.
When selecting a turbulence model, it is important to consider the flow regime. For example, if the flow is laminar, a turbulence model may not be necessary. However, if the flow is turbulent, a turbulence model is essential to accurately predict the flow behavior. For highly turbulent flows, a more complex turbulence model may be necessary.
The required accuracy is another important factor when choosing a turbulence model. If high accuracy is required, a more complex turbulence model may be necessary. However, if the required accuracy is low, a simpler turbulence model may suffice. It is important to note that more complex turbulence models require more computational resources and may take longer to run.
Geometry is also an important consideration when choosing a turbulence model. For example, if the flow is confined, a turbulence model that accounts for wall effects may be necessary. Additionally, if the geometry is complex, a more sophisticated turbulence model may be required to accurately capture the flow behavior.
Finally, computational resources must also be taken into account when selecting a turbulence model. More complex turbulence models require more computational resources and may not be practical for some applications. It is important to choose a turbulence model that balances accuracy with computational efficiency.
In summary, choosing the right turbulence model is essential to obtain accurate results in computational fluid dynamics simulations. The choice depends on factors such as the flow regime, required accuracy, geometry, and computational resources. It is important to choose a turbulence model that balances accuracy with computational efficiency.
When Are Turbulence Models Not Needed?
While turbulence modeling is a fundamental aspect of fluid dynamics modeling, there are certain scenarios where it may not be necessary. For instance, when the fluid flows in a laminar regime, turbulence models may not provide any significant contribution to the results. Laminar flow is characterized by smooth, predictable flow patterns with no turbulence or chaotic eddies. This type of flow is often observed in low-speed, low-viscosity fluids, such as air or water flowing through small pipes or channels.
Another scenario where turbulence models may not be necessary is when simulating flows that involve chemical reactions or phase change. In these cases, the priority may be placed on accurate descriptions of thermodynamics or phase behavior over turbulence modeling. For example, when modeling the flow of a fluid undergoing a phase change, such as water turning into steam, it is important to accurately capture the thermodynamic properties of the fluid at each stage of the process. Similarly, when modeling the combustion of a fuel, the priority may be placed on accurately modeling the chemical reactions and heat transfer processes, rather than turbulence.
It is also worth noting that turbulence models can be computationally expensive, especially for complex geometries or high Reynolds number flows. Reynolds number is a dimensionless parameter that characterizes the relative importance of inertial forces to viscous forces in a fluid flow. High Reynolds number flows, which are characterized by large-scale turbulent eddies and chaotic flow patterns, can be particularly challenging to simulate accurately. In these cases, simplified models or alternative techniques such as Large Eddy Simulation (LES) or Direct Numerical Simulation (DNS) may be more appropriate.
In summary, while turbulence modeling is a critical aspect of fluid dynamics modeling, it is not always necessary or appropriate. The choice of modeling approach depends on the specific flow regime, the physical phenomena of interest, and the computational resources available.
How Much Accuracy is Lost Because We Model the Impacts of Small Scale Turbulence?
The accuracy lost due to the modeling of small-scale turbulence depends on many factors. These include the modeling method, the size of the mesh, and the Reynolds number. In general, a higher Reynolds number leads to more extensive turbulence effects and requires smaller mesh sizes to capture the behavior accurately. Various studies showed that using turbulence models with meshes of reasonable size can accurately predict many flow characteristics.
However, it is important to note that small-scale turbulence can have a significant impact on the accuracy of the model. This is because small-scale turbulence can cause fluctuations in the flow that are difficult to capture with larger mesh sizes. These fluctuations can lead to errors in the model predictions, which can result in inaccurate results.
One approach to addressing this issue is to use high-resolution meshes that can capture the small-scale turbulence. However, this approach can be computationally expensive and may not be practical for all simulations. Another approach is to use advanced turbulence models that can account for the effects of small-scale turbulence. These models can help to improve the accuracy of the simulation results without requiring extremely fine meshes.
In addition to the modeling approach, the accuracy of the simulation results can also be impacted by the boundary conditions and the physical properties of the fluid being simulated. For example, the viscosity and density of the fluid can affect the behavior of small-scale turbulence and the accuracy of the simulation results.
Overall, while modeling the impacts of small-scale turbulence can lead to some loss of accuracy, there are various approaches that can be used to mitigate this issue. By carefully selecting the modeling approach and considering the physical properties of the fluid being simulated, it is possible to obtain accurate results even when modeling small-scale turbulence.
Examining the Different Types of Turbulence Models
Various turbulence models exist, each with its own set of assumptions and limitations. The zero-equation models are the most straightforward models to implement, while two-equation models provide the most comprehensive representation of the turbulence. Reynolds stress models provide a balance between accuracy and computational expense. Large-Eddy Simulation models are suitable for modeling flows with large scales of turbulence, while Detached Eddy Simulation models improve the prediction of separated flows. Choosing the appropriate model requires a thorough understanding of the available models' strengths and limitations.
Zero-equation models are the simplest turbulence models, and they are often used in engineering applications where computational resources are limited. They assume that the turbulent kinetic energy is proportional to the mean velocity, and the turbulence length scale is proportional to the characteristic length scale of the flow. While these models are easy to implement, they are not suitable for complex flows or flows with significant variations in turbulence intensity.
Two-equation models are the most widely used turbulence models in engineering applications. They provide a more comprehensive representation of the turbulence by solving two transport equations for the turbulent kinetic energy and the turbulence length scale. These models are suitable for flows with varying turbulence intensity and complex geometries. However, they require more computational resources than zero-equation models.
Reynolds stress models provide a balance between accuracy and computational expense. They solve for the Reynolds stresses, which are the fluctuations in velocity that cause turbulence. These models are suitable for flows with moderate to high levels of turbulence intensity and are often used in aerospace applications. However, they require more computational resources than zero-equation models and are less accurate than two-equation models.
Large-Eddy Simulation models are suitable for modeling flows with large scales of turbulence, such as those found in atmospheric flows and combustion applications. These models solve for the large turbulent structures explicitly and model the smaller scales of turbulence. They are computationally expensive and require high-resolution grids to capture the large turbulent structures accurately.
Detached Eddy Simulation models improve the prediction of separated flows, where the flow separates from a surface and creates a region of low-pressure turbulence. These models use a hybrid approach, where the large turbulent structures are resolved explicitly, and the smaller scales of turbulence are modeled. They are suitable for flows with moderate to high levels of turbulence intensity and are often used in automotive and aerospace applications.
In conclusion, choosing the appropriate turbulence model requires a thorough understanding of the available models' strengths and limitations. The choice of model depends on the complexity of the flow, the level of turbulence intensity, and the available computational resources. Zero-equation models are the most straightforward models to implement, while two-equation models provide the most comprehensive representation of the turbulence. Reynolds stress models provide a balance between accuracy and computational expense. Large-Eddy Simulation models are suitable for modeling flows with large scales of turbulence, while Detached Eddy Simulation models improve the prediction of separated flows.
Summary
In summary, turbulence modeling is a crucial aspect of fluid dynamics modeling. It provides a predictive tool for the behavior of fluids in various applications. Understanding the different types of turbulence models and their strengths and limitations is essential to choosing the correct model for a specific application.
One of the most commonly used turbulence models is the Reynolds-averaged Navier-Stokes (RANS) model. This model averages the velocity and pressure fields over time, resulting in a set of equations that can be solved numerically. However, RANS models are limited in their ability to accurately predict complex turbulent flows, such as those found in high-speed aerodynamics or turbulent boundary layers.
Another popular turbulence model is the large eddy simulation model. This model resolves the largest turbulent structures in the flow and models the smaller, unresolved structures. LES models are computationally expensive but can provide accurate predictions for complex turbulent flows.
Researchers continue to refine turbulence models and develop new methods to account for previously unmodeled phenomena. For example, the detached eddy simulation model combines aspects of both RANS and LES models to provide accurate predictions for flows with both attached and separated flow regions.
Additionally, advancements in computing power have allowed for the development of machine learning algorithms to improve turbulence modeling. These algorithms can learn from large datasets of flow simulations and provide more accurate predictions for complex flows.
In conclusion, while turbulence modeling has come a long way in providing accurate predictions for fluid flows, there is still much work to be done in refining and developing new models. With continued research and advancements in computing power, we can expect even greater predictive power for flow simulations in the future.