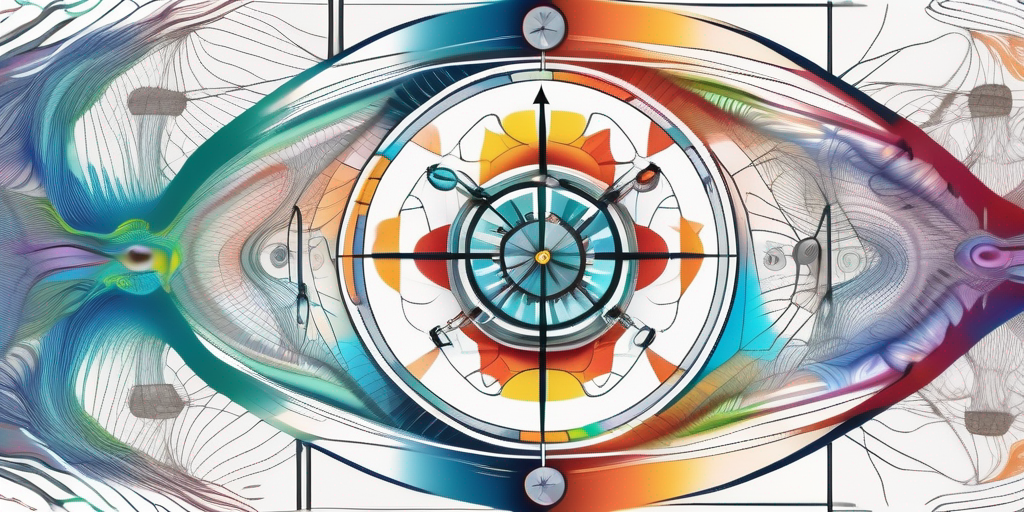
In Introduction to Data Post-Processing in CFD
Making Sense of Data from CFD Simulations
Computational Fluid Dynamics (CFD) simulations have become an integral part of the design and analysis process in various industries. By providing insights into the behavior of fluid flows, CFD simulations allow engineers and scientists to optimize designs, improve performance, and reduce costs. However, the data generated from these simulations can be complex and overwhelming. In this article, we will explore the basics of CFD simulations, the process involved, how to interpret the data, techniques to improve accuracy, and future trends in the field.## Understanding the Basics of CFD Simulations
What is Computational Fluid Dynamics?
Computational Fluid Dynamics, or CFD, is a computational tool used to simulate the behavior of fluids, such as air and water, in various physical systems. It applies mathematical equations, known as governing equations, to model fluid flows and their interactions with solids or other fluids.
CFD simulations involve dividing the fluid domain into a grid of small cells, known as a mesh. The governing equations are then solved numerically for each cell, taking into account factors such as fluid velocity, pressure, temperature, and turbulence. By solving these equations iteratively, CFD software can predict how the fluid will behave under different conditions.
CFD simulations can be used to analyze a wide range of fluid flow phenomena, including laminar and turbulent flows, compressible and incompressible flows, and steady-state and transient flows. This versatility makes CFD a powerful tool for studying fluid dynamics in various engineering applications.
Importance of CFD in Various Industries
CFD simulations are widely used in industries such as aerospace, automotive, energy, and manufacturing, to name a few. They provide valuable insights into aerodynamics, heat transfer, combustion, and multiphase flows. Engineers can study and optimize designs, assess performance, and minimize risks before physical prototypes are built, saving time and resources.
In the aerospace industry, CFD is crucial for designing efficient and aerodynamically stable aircraft. By simulating the airflow around the aircraft's wings, fuselage, and other components, engineers can identify areas of high drag or turbulence and make design modifications to improve fuel efficiency and reduce noise.
In the automotive industry, CFD is used to optimize the aerodynamics of vehicles, reducing drag and improving fuel economy. It is also employed in the design of cooling systems to ensure that engines and other components operate within safe temperature limits.
In the energy sector, CFD is used to study the behavior of fluids in power plants, such as nuclear reactors and gas turbines. By simulating the flow of coolant or working fluids, engineers can optimize the design and operation of these systems, improving efficiency and safety.
In the manufacturing industry, CFD is used to optimize the performance of various processes, such as mixing, heat transfer, and chemical reactions. By simulating fluid flows and their interactions with solid surfaces, engineers can identify areas of low or high fluid velocity, temperature gradients, or concentration gradients, enabling them to design more efficient and cost-effective processes.
Overall, CFD simulations play a crucial role in enhancing the understanding of fluid dynamics and enabling engineers to make informed decisions in various industries. By providing detailed insights into complex fluid behavior, CFD helps improve designs, optimize performance, and reduce risks, ultimately leading to more efficient and reliable products and processes.
The Process of CFD Simulations
The process of conducting a Computational Fluid Dynamics (CFD) simulation typically involves several steps. These steps are crucial in order to obtain accurate and reliable results. Let's take a closer look at each step:
Step 1: Problem Definition
The first step in a CFD simulation is to define the problem at hand. This includes clearly specifying the domain of interest, which refers to the physical space where the fluid flow is occurring. The boundary conditions, such as inlet and outlet velocities, temperature, and pressure, also need to be defined. Moreover, the required accuracy of the simulation must be determined, as this will influence the level of detail and complexity of the subsequent steps.
Step 2: Geometry Creation
Once the problem is defined, the next step is to create or import the geometry of the system into a CFD software. This involves constructing a digital representation of the physical system, including all the relevant components and their spatial arrangement. The geometry should accurately reflect the real-world system in order to obtain meaningful results.
Step 3: Physics Specification
After the geometry is established, the physics of the flow need to be specified. This includes defining the fluid properties, such as density, viscosity, and thermal conductivity. Additionally, the choice of turbulence model is crucial, as turbulence plays a significant role in many fluid flow scenarios. Selecting an appropriate turbulence model that accurately captures the flow characteristics is essential for obtaining reliable results.
Step 4: Simulation Execution
With the problem defined, geometry created, and physics specified, the simulation is ready to be executed. This involves solving the governing equations of fluid flow numerically using computational algorithms. The simulation software divides the domain into small computational cells and iteratively solves the equations to simulate the fluid flow behavior. The accuracy and convergence of the simulation depend on the chosen numerical methods and the computational resources available.
Step 5: Results Post-processing and Analysis
Once the simulation is completed, the results need to be post-processed and analyzed. This involves extracting relevant data, such as velocity profiles, pressure distributions, and temperature gradients, from the simulation output. Visualization techniques are often employed to better understand the flow behavior and identify any potential issues or areas for improvement. The results can be compared with experimental data or used for further analysis and optimization.
Common Challenges in CFD Simulations
Despite the advantages of CFD simulations, they come with their own set of challenges that need to be addressed. Some of the common challenges include:
- Mesh Generation: Creating a suitable mesh that accurately represents the geometry and captures the flow features is crucial. The mesh density, element types, and quality can significantly impact the simulation results.
- Turbulence Modeling: Selecting an appropriate turbulence model that can accurately capture the complex flow phenomena is a challenging task. Different turbulence models have different strengths and limitations, and their selection should be based on the specific flow conditions.
- Boundary Conditions: Accurately defining the boundary conditions is essential for obtaining meaningful results. Errors or uncertainties in specifying the boundary conditions can lead to inaccurate predictions of the flow behavior.
- Computational Resources: CFD simulations often require significant computational resources, including high-performance computing clusters and large memory capacities. Long computational times can be a limitation, especially for complex simulations with fine meshes and detailed physics models.
Overcoming these challenges requires expertise, experience, and careful considerations. CFD simulations should be conducted by knowledgeable professionals who can ensure the accuracy and reliability of the results.
Interpreting Data from CFD Simulations
Key Metrics in CFD Data
When interpreting CFD data, engineers typically focus on key metrics such as velocity profiles, pressure distributions, and heat transfer rates. These metrics provide insight into the behavior of the fluid and allow comparisons between different design variations or scenarios. Visualization techniques, such as contour plots and vector fields, aid in understanding the flow patterns and identifying areas of concern.
Tools for Analyzing CFD Data
Several software tools are available for analyzing CFD data. These tools enable engineers to extract important information, generate plots and graphs, and perform statistical analysis. They also facilitate the comparison of simulation data with experimental or theoretical data, validating the accuracy of the CFD models.
Improving Accuracy in CFD Simulations
Best Practices for Reliable Simulations
To improve the accuracy of CFD simulations, engineers should follow best practices. This includes conducting mesh sensitivity studies to ensure the mesh resolution is adequate, selecting appropriate turbulence models based on the flow conditions and objectives, and performing validation against experimental data. It is also crucial to consider the limitations and assumptions of the CFD models used.
Overcoming Common Errors in CFD Simulations
Errors can arise in CFD simulations due to various factors, such as inadequate grid resolution, improper boundary conditions, or numerical instabilities. Proper verification and validation processes, including solution verification and grid convergence studies, can help identify and minimize these errors. Additionally, understanding the physics of the flow and the limitations of the CFD software used is essential to produce reliable and trustworthy results.
Future Trends in CFD Simulations
Technological Advancements Impacting CFD
As technology continues to advance, CFD simulations are benefiting from innovations in areas such as computer hardware, numerical algorithms, and optimization techniques. High-performance computing clusters, improved turbulence models, and sophisticated techniques for uncertainty quantification are enabling more accurate and efficient simulations. These advancements are expanding the capabilities of CFD and broadening its applications.
The Role of AI and Machine Learning in CFD Simulations
The integration of AI and machine learning techniques with CFD simulations is a promising trend. These technologies can assist in automating certain aspects of the simulation process, optimizing designs, and predicting flow behavior. Machine learning algorithms can also be used to analyze large datasets generated from CFD simulations, extracting patterns and insights that may not be easily discernible using traditional techniques.
In conclusion, CFD simulations provide a powerful tool for understanding and optimizing fluid flows in various industries. By following best practices, interpreting key metrics, and leveraging advanced techniques, engineers can make sense of the data generated from CFD simulations, leading to improved designs, enhanced performance, and cost savings. With the ongoing advancements in technology and the integration of AI and machine learning, the future of CFD simulations looks promising, opening up new possibilities for innovation and optimization.